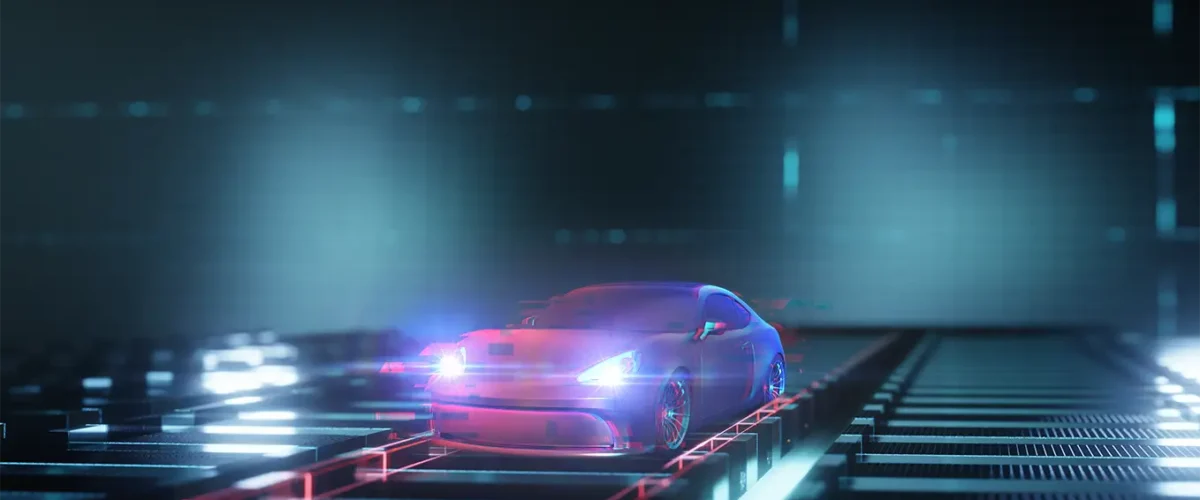
A.D.L Data Lifecycle Solution in AD/ADAS
In the context of autonomous driving, the Data Lifecycle refers to the process of collecting data from various sensors on testing vehicles or production vehicles, annotating the collected data, training neural network models with annotated data in the cloud, and then deploying the trained models to vehicles via over-the-air (OTA) updates.
Data Lifecycle is essential for autonomous driving and ADAS development.
Solving corner-case problem
For any L2/L2+ ADAS system, continuous evolution capability is mandatory. To ensure this goal, it is necessary to continually collecting data on corner cases. Rule-based AD/ADAS is turned into End-to-End AI based method, makes data-driven development become more important.
Model Generalization
Currently, ADAS is making strides from highway scenarios into urban scenarios. Solving relatively simple scenarios like highways typically requires training models primarily based on data collected from test vehicles, without necessarily needing to transmit data from production vehicles. However, the complexity of urban environments has significantly increased, with various differences in road conditions across different cities.
To achieve the goal of scene interoperability, the model’s generalization capability needs to be significantly enhanced. Improving the generalization capability of the model requires collecting data corresponding to various scenarios as comprehensively as possible.
Driving AI Model Iteration
The development of AI based on deep learning has surpassed a decade. During this period, with the evolution of models and the advancement of compute capability, the ability of autonomous driving systems to digest big data has become feasible. Furthermore, for autonomous driving systems to upgrade, improvements in perception, planning, and other aspects are necessary. Adopting a data-driven approach allows algorithms to continuously evolve, which proves to be an efficient means of enhancing capabilities in perception, planning, and other aspects.
Challenges in AD/ADAS Data Lifecycle Approach
« Sensor Complexity
With the proliferation of sensors such as LiDAR, radar, cameras, and GNSS/INS, autonomous vehicles are equipped with a diverse array of data sources to perceive their surroundings. However, this abundance of sensors poses several significant challenges.
« System Throughput
Due to the substantial volume of data recorded during the logging session, significant system bandwidth will be consumed. Moreover, ensuring the accuracy and reliability of time synchronization across the entire system presents another challenging aspect.
Check Simplined ADL Data Logging Station for a high performance and robust solutions.
« S/W Tool-Chain
To enhance the efficiency of the data-driven development, an efficient and convenient s/w tool-chain is indispensable. A streamlined and user-friendly toolchain enables the efficient utilization of data to drive model updates. Moreover, it facilitates objective quantification of research and development accomplishments, thereby expediting the iteration process of autonomous driving systems.
Check out Simplined Data-logging s/w tool-chain
We are heading to
The future of AD/ADAS Data Lifecycle
Cloud Computing empowered Data logging
Cloud computing has revolutionized data logging in the realm of autonomous driving. By leveraging cloud infrastructure, data logging processes can now be significantly empowered.f
Auto-Labeling and Ground Truth
Auto-labeling involves using algorithms or machine learning models to automatically annotate or label sensor data with relevant information. This process is essential for efficiently handling large volumes of data, as manual labeling can be time-consuming and impractical. Auto-labeling algorithms can classify objects, detect road markings, identify traffic signs, and more, providing valuable context to the logged data.
Ground truth refers to the accurate and reliable reference data used to evaluate the performance of autonomous driving systems. Ground truth data is crucial for training and validating machine learning models, assessing the performance of perception algorithms, and benchmarking the accuracy of autonomous driving systems. Ensuring the accuracy and consistency of ground truth data is essential for reliable evaluation and improvement of autonomous driving technology.
Simulation and Digital Twins
Simulation platforms offer virtual testing environments where algorithms can be thoroughly evaluated under diverse scenarios, including rare edge cases and adverse weather conditions. They provide scalability, cost-efficiency, and enable iterative development by rapidly generating large volumes of data. Digital twins, on the other hand, are virtual representations of physical objects or systems, continuously updated to reflect real-world changes. They facilitate predictive analytics, remote monitoring, and maintenance, offering insights into performance and enabling proactive interventions. Together, simulation and digital twins accelerate the development and deployment of autonomous driving systems while ensuring safety, reliability, and efficiency.
Send Us A Message
Get In Touch
Address
NO.72-76 WING LOK STREET, SHEUNG WAN, HONG KONG
Email (Global)
Email (Japan)
Phone
+852 9297 9555